FAIR pratices for HPC's ML models and datasets
Findable HPC Data
We provide a GraphQL interface for the high-level data using a common software stack (Graphene/MongoEngine/MongoDB). We use BlazeGraph to store the all data, submitted high-level metadata and processed data file (such as CSV). These data can be queried using SparQL.
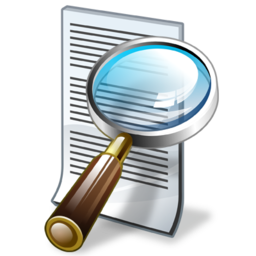
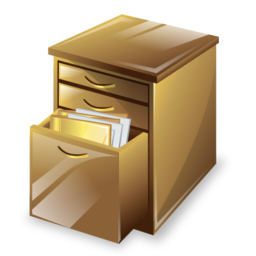
Accessible HPC Data
RestAPI, GraphQL, SparQL, ...
Interoperable HPC Data
We designed a two-levels ontology. The high-level ontology permits analyst to model the HPC environment (projects, hardware, software, ...). The low-level ontology is used to model measurement performed on HPC software and hardware.
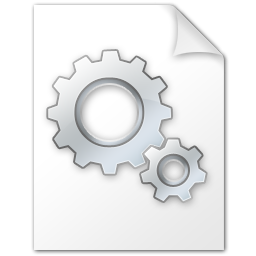
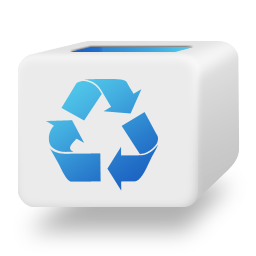
Reusable HPC Data
Our high-level ontology permit contributors to describe their HPC experiments, data processing steps, AI model training, and much more.